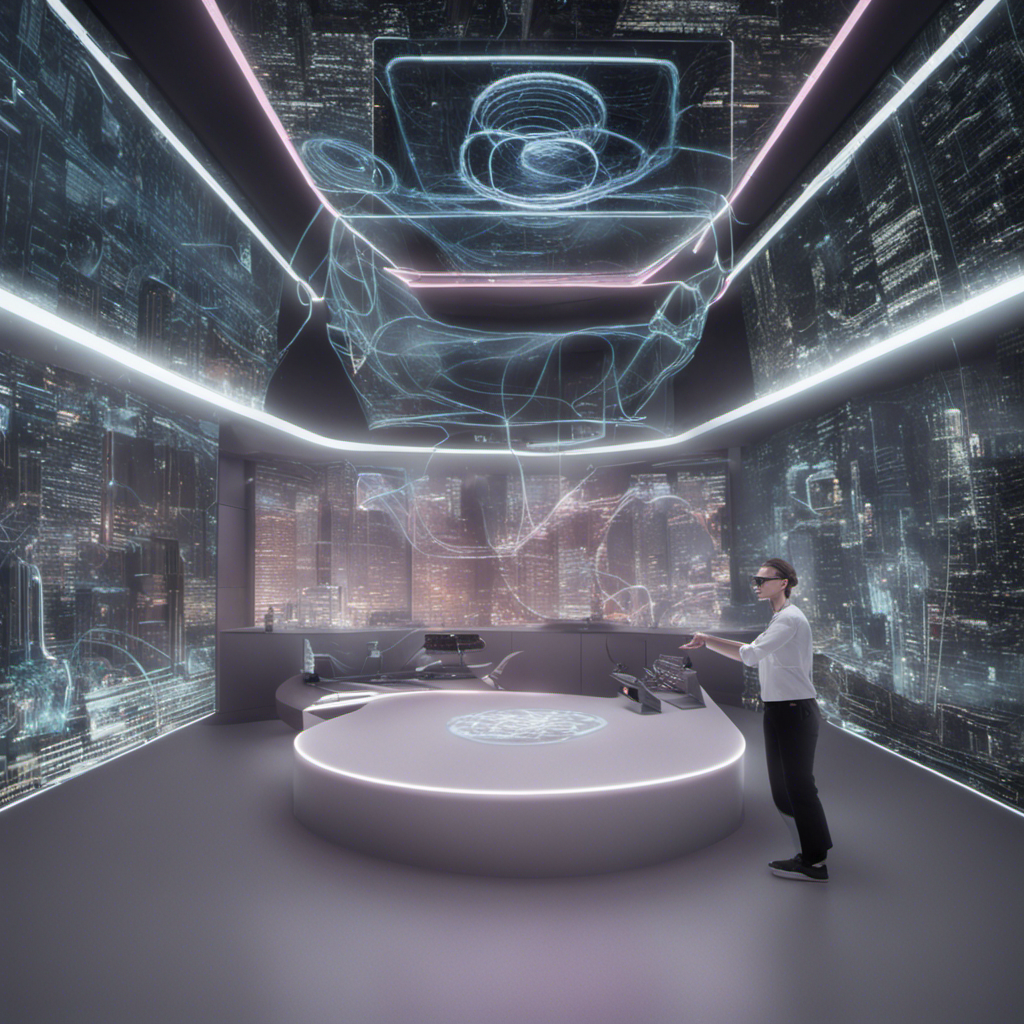
Introduction to Data Science: Analysis and Visualization
From predictive analytics to complex modeling, data science has gained significant traction in the popular imagination. In a digital age thriving on information, data science stands as a pillar of technological progress and prosperity. As with any technology, the journey to becoming a proficient Data Scientist begins with understanding the basics. This blog post aims to be a comprehensive introduction to Data Science, with special focus on Analysis and Visualization. We’ll delve into the immense potential of data, learn about the tools and methodologies for analysis and visualization, and witness first-hand how data can tell a compelling story.
What is Data Science?
In its simplest form, Data Science is a fusion of various disciplines that work in unison to extract insights from data. Fields such as mathematics, statistics, and computer science are at the forefront of this multi-disciplinary approach.
The Harvard Business Review termed Data Science as “The sexiest job of the 21st century.” But why? Simply put, it’s because of the enormous value Data Scientists bring to the organizations by exploring complex datasets to make informed business decisions1.
The Two Pillars - Analysis and Visualization
Understanding data is a two-step process: Analysis and Visualization. They are the heart and soul of Data Science.
Data Analysis
Data Analysis is the process of cleaning, inspecting, transforming, and modeling data with the goal of discovering useful information. The process commences with the extraction of raw data, which is subsequently cleaned to weed out any inconsistencies. Next, Exploratory Data Analysis2 (EDA) investigates the main characteristics of the data using visual and quantitative methods.
Data Visualization
Data Visualization, on the other hand, is the presentation of data in a graphical or pictorial format. With Visual analytics, we are better equipped to make sense of large data sets. We can spot patterns, trends, and correlations that might escape the normal, numerical analysis.
Tools of the Trade
Data Science is powered by various crucial tools that make the process of data analysis and visualization as smooth as butter. Some universally accepted tools include:
- Python and R for data manipulation and analysis
- Tableau and PowerBI for data visualization
- SQL for data extraction
- Jupyter Notebooks for creating and sharing codes, equations, visualizations, etc.
The Journey Ahead
Data science continues to evolve and innovate rapidly, acting as a catalyst for change in countless industries. With a solid understanding of data analysis and visualization, you’re now on the firm ground to build upon.
By mastering intricate analytical concepts and visualization techniques, you can bring a plethora of insights and improvements to any data-driven project. The journey may seem daunting, but the results are undoubtedly worth it. Let’s keep exploring!