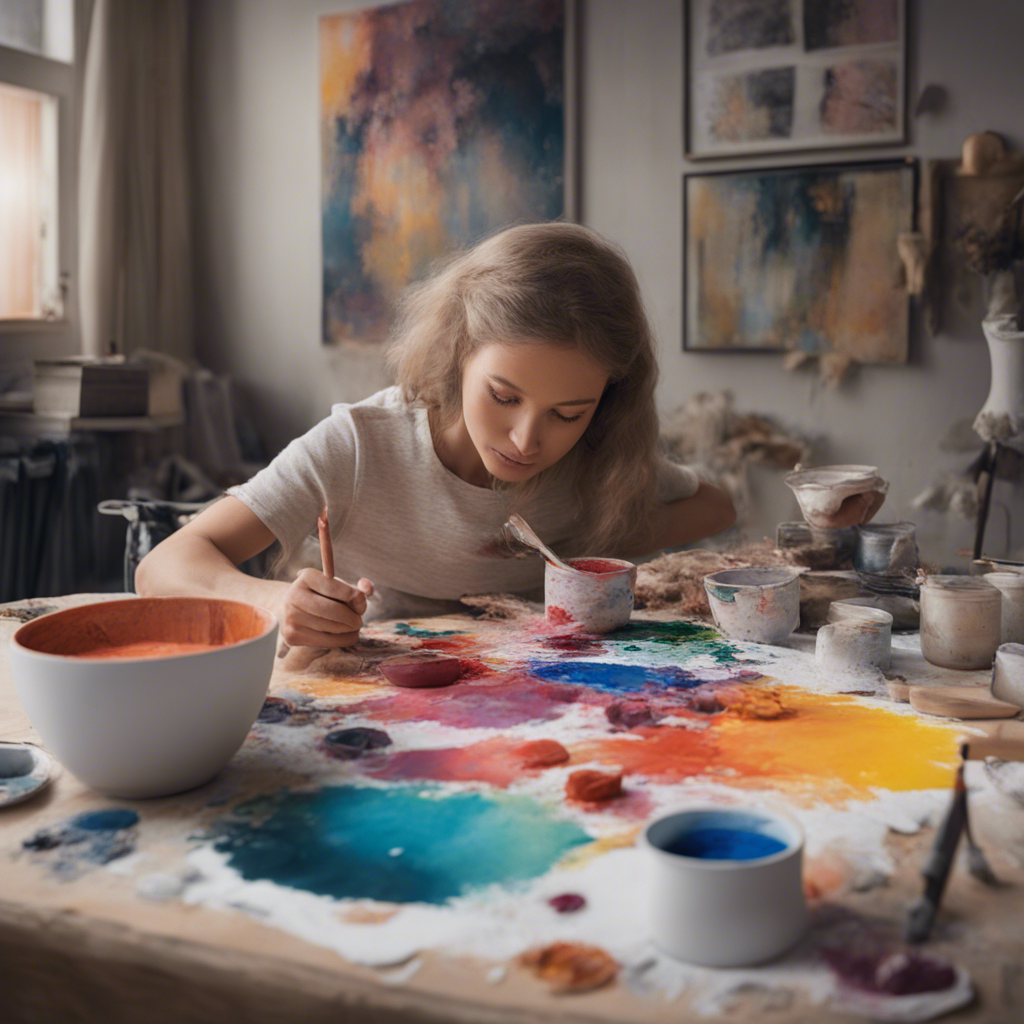
Generative AI: How Models Like DALL·E 2 Work
Introduction
Artificial intelligence (AI) has made significant strides in recent years, and one particularly fascinating area is generative AI. Generative AI refers to the development of algorithms and models capable of creating original content, such as images and text. One such model that has gained considerable attention is DALL·E 2. In this blog post, we will explore how models like DALL·E 2 work, their underlying architecture, and their potential applications.
Understanding Generative AI
Generative AI models go beyond traditional AI systems, which are primarily designed for classification or predictive tasks. These models are capable of generating completely new content that resembles the training data they were exposed to. Instead of relying on predefined rules or templates, generative AI models generate content by learning the patterns and structures of the data they were trained on.
The Architecture of DALL·E 2
DALL·E 2 (short for “Diverse Aspects of Forms Learned without Explicit 2D Supervision”) is a generative AI model developed by OpenAI. It builds upon the success of its predecessor, DALL·E, and utilizes state-of-the-art techniques in deep learning and natural language processing (NLP). Let’s dive into the architecture of DALL·E 2 to gain a deeper understanding.
Transformer-based Architecture
DALL·E 2 is built upon a transformer-based architecture, specifically a variation of the well-known transformer model called the GPT (Generative Pretrained Transformer). The transformer model is particularly adept at handling sequential data, making it suitable for generating coherent and contextually relevant content.
Multimodal Training
DALL·E 2 is trained on a massive dataset containing text and image pairs, allowing it to understand the underlying relationships between visual and textual elements. This multimodal training enables the model to generate images based on textual descriptions and vice versa, opening up a wide range of creative possibilities.
Pretraining and Fine-Tuning
Like many other generative AI models, DALL·E 2 undergoes two key stages: pretraining and fine-tuning. During the pretraining phase, the model is exposed to a large dataset containing diverse sources of text and images, enabling it to learn the statistical patterns and semantic relationships in the data. In the fine-tuning phase, the model is trained on a more specific dataset, further refining its ability to generate contextually appropriate content.
Applications of DALL·E 2
The capabilities of DALL·E 2 extend far beyond its impressive architecture. This model has the potential to revolutionize various industries and domains, including:
Creative Content Generation
DALL·E 2 can be used to generate unique and diverse images based on textual prompts. This has significant implications for artists, designers, and creatives seeking new sources of inspiration. By providing a textual description, such as “a cat flying through space wearing a top hat,” DALL·E 2 can generate imaginative and visually appealing images that serve as a starting point for further artistic exploration.
Data Augmentation
In fields where large, labeled datasets are essential, such as computer vision and natural language processing, DALL·E 2 can be employed to augment existing datasets. By generating synthetic but realistic data, this model can help increase the diversity and generalization of training data, ultimately improving the performance of downstream AI models.
Interactive Interfaces and Storytelling
DALL·E 2’s ability to generate images based on textual prompts opens up exciting possibilities for interactive interfaces and storytelling. Imagine a game where the player’s input influences the visual elements of the game world, or a chatbot that generates relevant visual content in real-time during a conversation. DALL·E 2’s generative capabilities have the potential to create immersive and engaging experiences in various interactive mediums.
Conclusion
Generative AI models like DALL·E 2 represent a significant breakthrough in artificial intelligence. Through deep learning and multimodal training, these models possess the ability to generate original and contextually relevant content. Their potential applications, ranging from creative content generation to data augmentation and interactive interfaces, open up new avenues for innovation across a wide range of industries. As research in generative AI continues to progress, we can expect even more astonishing developments in the future.
*References: