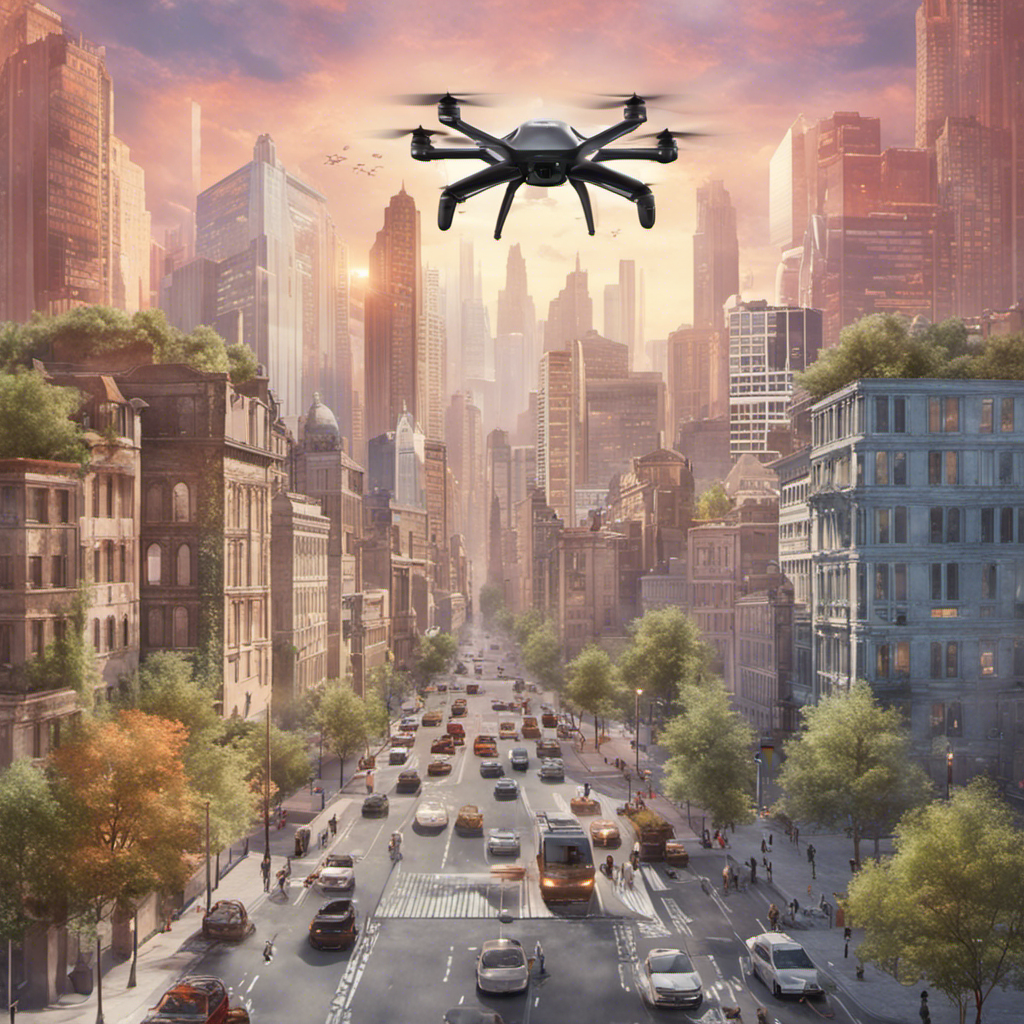
Practical Applications of Reinforcement Learning
Reinforcement learning, a subset of artificial intelligence (AI), has gained significant attention in recent years due to its ability to train intelligent algorithms to make decisions and take actions based on trial and error interactions with an environment. While it initially gained popularity in game playing scenarios, reinforcement learning has since found numerous practical applications across a wide range of industries. In this blog post, we will explore some of the most compelling practical applications of reinforcement learning and the impact it has had on various domains.
Robotics and Automation
Reinforcement learning has revolutionized the field of robotics and automation by enabling robots to learn tasks through repeated interactions with their environment. This has led to significant advancements in autonomous systems, enabling robots to perform complex actions in dynamic and unstructured environments. For example, robots can now learn to navigate through cluttered spaces, perform object manipulation tasks, and even learn to walk. Reinforcement learning allows these robots to adapt and improve their performance over time, making them more efficient and capable.
Healthcare
The application of reinforcement learning in healthcare has the potential to greatly enhance medical diagnosis, treatment, and patient care. Machine learning algorithms can be trained using reinforcement learning to analyze patient data, such as electronic health records and medical images, to assist in disease diagnosis and personalized treatment planning. Additionally, reinforcement learning can be used to optimize treatment protocols and drug dosages, leading to more effective and personalized healthcare solutions.
Finance and Trading
Reinforcement learning has shown promising results in the field of finance and trading, where decision-making under uncertain conditions is crucial. By training algorithms to learn optimal trading strategies through trial and error, reinforcement learning can help financial institutions and traders make better investment decisions and manage risk more effectively. Furthermore, reinforcement learning techniques can be used to develop algorithmic trading systems that adapt to changing market conditions, leading to higher returns on investments.
Transportation
With the rise of autonomous vehicles, reinforcement learning has become a powerful tool for training these vehicles to navigate safely and efficiently on the roads. By allowing autonomous vehicles to learn from their experiences and make decisions based on real-time data, reinforcement learning enables them to respond and adapt to changing traffic conditions. Furthermore, reinforcement learning can be used to optimize traffic control systems, leading to smoother traffic flow and reduced congestion.
Natural Language Processing
Reinforcement learning has also made significant contributions to the field of natural language processing (NLP). By applying reinforcement learning algorithms to NLP tasks, such as machine translation and dialogue generation, we can enhance the ability of machines to understand and generate human language. Reinforcement learning can be used to improve language models, making them more accurate and capable of understanding context, leading to more effective communication between humans and machines.
Gaming and Entertainment
One of the earliest areas where reinforcement learning gained prominence is in gaming and entertainment. By training intelligent agents to learn optimal strategies through trial and error, reinforcement learning has resulted in groundbreaking advancements in game playing. For instance, AlphaGo, a reinforcement learning-based AI program, defeated world champions in the ancient game of Go. This showcases the potential of reinforcement learning for solving complex problems and pushing the boundaries of AI capabilities in various forms of gaming and entertainment.
Conclusion
Reinforcement learning has emerged as a powerful technique with practical applications spanning multiple industries. From robotics and healthcare to finance and gaming, reinforcement learning has enabled significant advancements and has the potential to transform the way we interact with technology in various domains. As the field continues to evolve, we can expect to see even more innovative applications that will shape the future of AI and its impact on our daily lives.
References:
- Silver, D. et al. (2017). Mastering the game of Go without human knowledge. Nature, 550(7676), 354-359. [doi:10.1038/nature24270]
- Sutton, R. S., & Barto, A. G. (2018). Reinforcement Learning: An Introduction. MIT Press.
- Li, L., et al. (2021). Reinforcement Learning for Autonomous Vehicles: A Systematic Literature Review. Applied Sciences, 11(3), 1228. [doi:10.3390/app11031228]