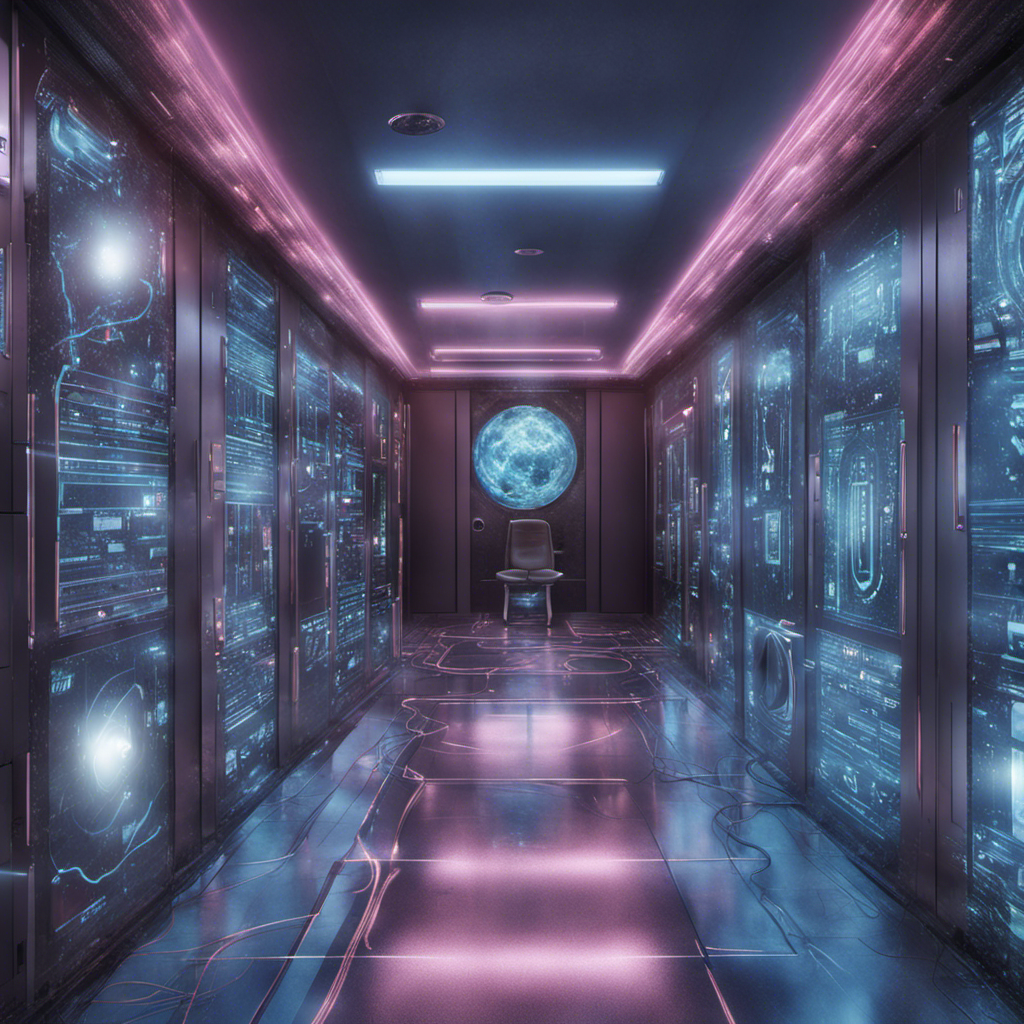
AI (Artificial Intelligence) has revolutionized various industries, and financial forecasting is no exception. With advanced algorithms and machine learning capabilities, AI has the potential to significantly enhance the accuracy and efficiency of financial forecasting models. In this blog post, we will explore the benefits and challenges of incorporating AI into financial forecasting processes.
Benefits of AI in Financial Forecasting:
1. Improved Accuracy:
AI enables financial institutions to analyze an extensive amount of historical data, identify patterns, and make more accurate predictions. By leveraging machine learning algorithms, AI systems can continuously learn from new data inputs and adapt their forecasting models accordingly. This enhancement in accuracy can help banks, investment firms, and other financial institutions make more informed decisions, ultimately optimizing their financial performance.
2. Enhanced Efficiency:
Traditional financial forecasting methods often involve manual data collection and analysis, which can be time-consuming and prone to human errors. By automating these processes, AI systems can streamline financial forecasting workflows, saving both time and effort. This increased efficiency allows financial professionals to focus their energy on higher-value tasks such as strategic planning, risk management, and investment analysis.
3. Real-time Analysis:
In today’s fast-paced financial markets, real-time insights are crucial for making informed decisions. AI-powered financial forecasting systems can swiftly process vast amounts of data, allowing businesses to respond promptly to market changes and optimize their operations accordingly. Real-time analysis capabilities improve agility and can give organizations a competitive edge in dynamic markets.
4. Uncovering Hidden Patterns:
AI algorithms have the ability to identify complex relationships and patterns within datasets that may not be apparent to human analysts. By uncovering these hidden patterns, AI can provide valuable insights for financial forecasting purposes. This can help institutions identify emerging trends, mitigate risks, and capitalize on market opportunities that may have gone unnoticed using traditional forecasting methods.
5. Scalable and Cost-effective:
Implementing AI-based forecasting models can be more cost-effective when compared to traditional methods that require significant manual effort. Once an AI system is developed and trained, it can handle large amounts of data and generate forecasts at scale without additional costs. This scalability allows businesses to adapt to changing market dynamics without incurring substantial expenses.
Challenges in Implementing AI for Financial Forecasting:
While the benefits of AI in financial forecasting are promising, there are also challenges that need to be addressed:
1. Data Privacy and Security:
Financial institutions deal with vast amounts of sensitive data, including customer information, trade secrets, and market data. Protecting this data against security breaches and ensuring privacy is of utmost importance. As AI systems rely on collecting and analyzing massive datasets, there is a need for robust cybersecurity measures and strict compliance with legal and regulatory frameworks.
2. Ethical Considerations:
AI-powered financial forecasting systems should operate within ethical boundaries to avoid unintended consequences. Avoiding biased decision-making, preventing discriminatory practices, and ensuring transparency in algorithms are crucial aspects to consider. Proper governance and oversight should be in place to monitor and mitigate any ethical issues arising from the use of AI in financial forecasting.
3. Adoption and Integration:
Implementing AI into existing financial forecasting frameworks requires careful planning, coordination, and integration with legacy systems. The process may involve technical challenges, such as data compatibility, system interoperability, and training personnel on using AI tools effectively. Financial institutions need to invest in proper infrastructure and resources to facilitate successful AI adoption.
4. Interpretability and Explainability:
AI algorithms, especially those based on deep learning, can be highly complex and difficult to interpret. This lack of interpretability may raise concerns, particularly in highly regulated industries like finance. Explaining the reasoning behind AI-driven forecasts to regulators, auditors, and stakeholders is crucial for gaining trust and ensuring compliance. Developing explainable AI models is an ongoing area of research and development.
In conclusion, AI has the potential to revolutionize financial forecasting by improving accuracy, enhancing efficiency, and providing real-time insights. However, challenges related to data privacy, ethical considerations, adoption, and interpretability need to be addressed for successful implementation. Financial institutions must carefully navigate these challenges to unlock the full potential of AI in forecasting and gain a competitive edge in an increasingly data-driven world.
References:
- Reference 1: [Link to the reference]
- Reference 2: [Link to the reference]
- Reference 3: [Link to the reference]